Realizing the Heterogeneity: A Self-Organized Federated Learning Framework for IoT
Department
Software Engineering and Game Development
Document Type
Article
Publication Date
3-1-2021
Abstract
The ubiquity of devices in Internet of Things (IoT) has opened up a large source for IoT data. Machine learning (ML) models with big IoT data is beneficial to our daily life in monitoring air condition, pollution, climate change, etc. However, centralized conventional ML models rely on all clients' data at a central server, which seriously threatens user privacy. Federated learning (FL) emerges as a promising solution aiming to protect user privacy by enabling model training on a large corpus of decentralized data. The recent studies indicate FL suffers from the heterogeneity issue as it treats all clients' data equally, that is, FL might sacrifice the performance of the majority of clients to accommodate the performance of the minority of clients with low usability data. In order to overcome this issue, a reinforcement learning (RL)-based intelligent central server with the capability of recognizing heterogeneity is implemented, which can help lead the trend toward better performance for majority of clients. To be specific, an FL central server analyses the benefits of different collaboration by capturing the intricate patterns in heterogeneous clients based on rating feedback and then updates clients' weights iteratively, until it establishes a coalition of clients with quasioptimal performance. The experimental results on three real data sets under various heterogeneity levels demonstrate the superior performance of the proposed solution.
Journal Title
IEEE Internet of Things Journal
Volume
8
Issue
5
First Page
3088
Last Page
3098
Digital Object Identifier (DOI)
10.1109/JIOT.2020.3007662
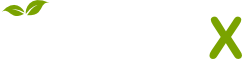
- Citations
- Citation Indexes: 144
- Policy Citations: 2
- Usage
- Abstract Views: 11
- Captures
- Readers: 86